FATE MORPH
These comments provided by Neurotechnology are based on NIST FATE MORPH ongoing evaluation results reviewed on December 20, 2023.
NIST FATE MORPH rigorously measures the effectiveness of algorithms designed to detect face morphing attacks. These attacks combine multiple faces into a single-face image to potentially compromise identity verification processes. Such manipulations can fool human checks and automated face recognition systems.
This evaluation employs a diverse collection of datasets, encompassing a variety of morphing techniques ranging from those accessible to the general public, like web-based services or paper crafts, to sophisticated methods used in academic research and commercial applications. The assessment follows a tiered approach, examining technology performance across a spectrum of morphs, from those created with non-expert tools to those produced with advanced software.
See FATE official page for more information.
The evaluation uses two main key metrics: the Attack Presentation Classification Error Rate (APCER), which is the rate of missed morphs, and the Bona Fide Classification Error Rate (BPCER), which is the rate of false detections. While aiming for low false detection rates is crucial to avoid expending resources on verifying legitimate photos, even an automated system with zero false detections but higher morph miss rates represents an operational improvement over no morph detection.
There was a total of 48 algorithm submissions from different vendors.
The chart below shows the cross-dependence between these metrics for different experiments. The performance of the Neurotechnology algorithm submission is highlighted.
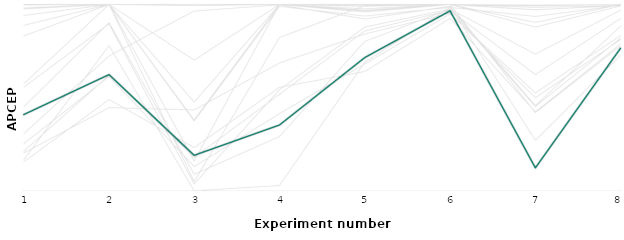
The horizontal axis on the chart corresponds to the performed experiments, which are described below:
- Local Morph Colorized Match, single image (BPCER=10%)
- Local Morph Colorized Match, single image (BPCER=1%)
- VISA-BORDER, single image (BPCER=10%)
- VISA-BORDER, single image (BPCER=1%)
- Manual, single image (BPCER=10%)
- Manual, single image (BPCER=1%)
- Print + Scanned, single image (BPCER=10%)
- Print + Scanned, single image (BPCER=1%)
Local Morph Colorized Match, single image
This experiment focuses on averaging only the face area of subjects after alignment and feature warping.
Neurotechnology algorithm showed these results:
- 40.9% APCER at 10% BPCEP. The most accurate contender showed 3.0% APCER at the same BPCEP.
- 62.4% APCER at 1% BPCEP. The most accurate contender showed 25.9% APCER at the same BPCEP.
The experiment used 1346 morphs and 254 source images, with 512x768 pixels image size. The morphs are generated through automated methods, consistently involving two subjects. In these morphs, both subjects contribute equally, with a subject alpha value of 0.5. Subject A provided the periphery, and the Face area is adjusted to match Subject A's color histogram. The morphs in this dataset used subjects of the same sex and ethnicity labels. The probe images used for evaluating differential Morphing Attack Detection are high-quality portrait images.
VISA-BORDER, single image
The experiment used visa-like images from a global population, and border crossing photos collected with a webcam of travelers entering the United States.
Neurotechnology algorithm showed these results:
- 19.0% APCER at 10% BPCEP. The most accurate contender showed 0.0% APCER at the same BPCEP.
- 35.3% APCER at 1% BPCEP. The most accurate contender showed 2.9% APCER at the same BPCEP.
The experiment used 25727 morphs and 51454 source images. The morphs are generated through automated methods, consistently involving two subjects. In these morphs, both subjects contribute equally, with a subject alpha value of 0.5. The morphs were made using subjects of matching age, gender, and nationality labels. The border-crossing photos frequently show differences in pose angles and lighting.
Manual, single image
The experiment used high-quality portrait images as probes to assess differential Morphing Attack Detection
Neurotechnology algorithm showed these results:
- 71.5% APCER at 10% BPCEP. The most accurate contender showed 4.9% APCER at the same BPCEP.
- 96.6% APCER at 1% BPCEP. The most accurate contender showed 35.7% APCER at the same BPCEP.
The experiment used 323 morphs and 825 source images, with 640x640 or 1080x1080 pixels image size. Morphs are generated using manual techniques with commercial software tools, equally blending two subjects where each contributes 50%. The image's label indicates the data provided to the software during processing from a specific dataset.
Print + Scanned, single image
The experiment used a subset of the images used in the Visa-Border experiment, which were printed on photo paper, and then scanned.
Neurotechnology algorithm showed these results:
- 12.3% APCER at 10% BPCEP. The most accurate contender showed 1.2% APCER at the same BPCEP.
- 76.3% APCER at 1% BPCEP. The most accurate contender showed 16.9% APCER at the same BPCEP.
The experiment used 3604 morphs and 2739 source images, with 600x600 pixels image size. Morphs are generated using manual techniques with commercial software tools, equally blending two subjects where each contributes 50%. The image's label indicates the data provided to the software during processing from a specific dataset. The live probe images used are border crossing photographs captured with a webcam of travelers entering the United States, and they can exhibit variations in pose angles and illumination.